Why Enterprise AI Adoption is Harder Than Expected
Jim Liddle discusses why AI strategy adoption is harder than expected within many large enterprise organizations.
December 10, 2024
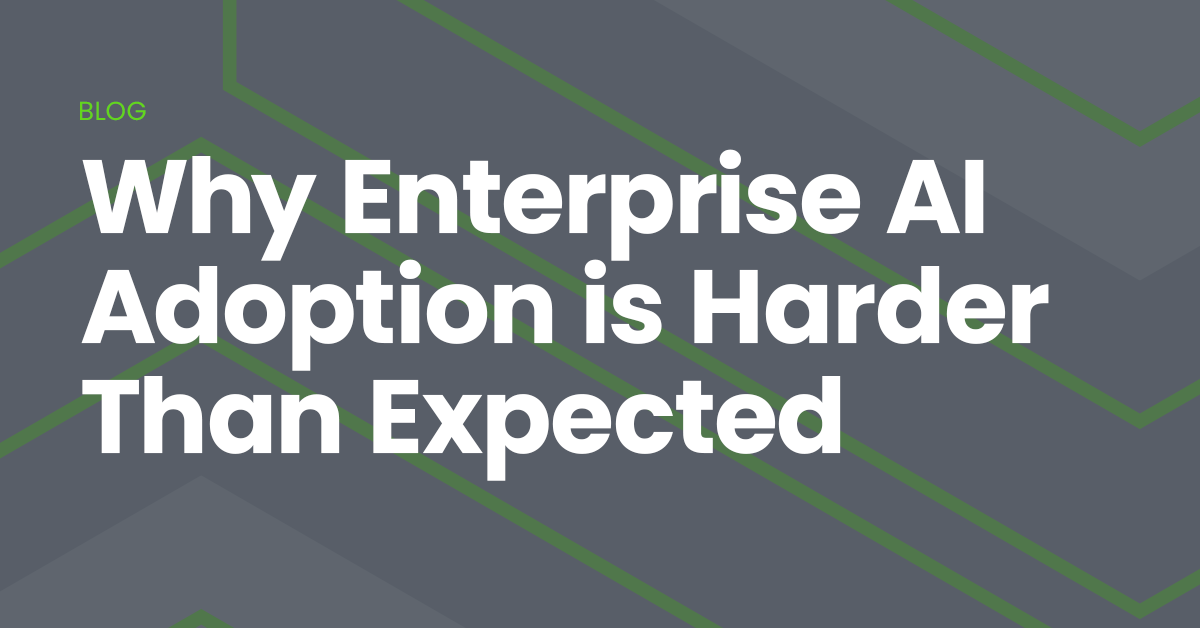
Over a recent weekend I was sorting out a drawer in my office when I found an old plug. Which gadget did it belong to? I hadn’t a clue, so I snapped a quick picture with my smartphone, sent it over to Google Gemini’s LLM, and I received all the relevant details about the mystery item within seconds. I was amazed, but I should not have been. Such capabilities are now expected. The progress we’ve seen since the release of ChatGPT just two years ago has been staggering. Consumer adoption is soaring. The tools are remarkably easy to use and undeniably powerful. Yet AI strategy adoption lags within many large enterprises. Why? There are four main reasons.
1. The space is moving too quickly
New models are being released all the time. A few weeks after one of the leading LLM vendors releases a model that outperforms the rest, a competitor puts out a newer model that’s even better. The models themselves are evolving in functionality, too. ChatGPT now incorporates voice and image generation, for example, and many other models are also now multimodal.
2. The security risks are real
It’s easy for me to take a picture of a plug and send it off to the model of my choice. There’s no security or data loss risk. A well-run enterprise, on the other hand, has to vet any new technologies. Your CISO and security operations teams must control the risk of deploying these advanced systems, especially if they have access to your company’s data.
3. The use cases aren’t obvious
The wide range of capabilities broadens the potential use cases but also makes identifying the right ones for your business extremely difficult. For example, I met recently with one of our AEC customers and showed them how they could use an AI strategy to analyze video of one of their job sites and spot potential code violations. This wasn’t an advertised function — it required creative thinking and understanding of the broad capabilities of one of the models.
4. The tools are expensive
Some of the resistance to enterprise adoption is purely commercial. A thousand employees at $30 per user per month for a leading AI tool is not chicken feed. If leadership are going to authorize that kind of spending, they are going to want measurable value. This is going to be a complex calculation, however, as it’s not a one-for-one replacement. The soft or hard-to-measure benefits may be the most powerful. The tools have the potential to make your people more productive, more efficient, and ultimately happier by offloading some of the drudgery they do on their own today.
5 Truths About Enterprise AI Adoption
So how should enterprises respond? I would not advise burying your head in the sand and pretending these technologies are going to go away. There is undoubtedly an excess of hype, but the core technology is undeniably powerful and getting more useful every week. Here are a few points to keep in mind as you move ahead with your strategic planning:
1. Data + AI ≠ Magic
Many enterprises are inclined to develop or fine-tune models in house, but the reality of implementing AI strategy within the enterprise is highly complex, involving data engineering, data science, and more. It is very difficult and time consuming to go from demo to production, and if you are trying to do this in a company with 1,000+ people, you are going to run into multiple unexpected issues. Those magical outcomes will prove elusive.
2. Your people already use AI
Never mind your policies. Many enterprises have issued blanket bans of AI tools for entirely valid security reasons, but my informal polling suggests that most employees are using them anyway. Instead of approving a select number of tools and fostering a more secure environment, you’re effectively promoting shadow IT and increasing your security risks by instituting a blanket ban.
3. Security buy-in is paramount
With that in mind, one of my primary pieces of advice for our customers, when we meet to discuss AI implementation, is to put together an AI Strategy Board that includes security and legal stakeholders. You need to work alongside these groups to sharpen your vision and plan for implementing enterprise-approved AI solutions — and to clarify the ramifications for those who disregard those rules and engage in shadow IT.
4. Expected value is complex
There are powerful competitive reasons for advancing AI use within your organization, as you risk falling behind your competitors if you do not. But the value proposition argument should begin with how your users — and your organization as a whole — will benefit from specific AI tools. If you have not mapped out those possibilities, ask your employees. They probably have a very clear idea of how these tools could help them in their day-to-day work.
5. You can’t go wrong with a hyperscaler
At this point, developing a custom model may be a waste of time considering the rate of improvement with commercial models. Instead of spending the next two years on an in-house project, you will likely find that the specifications you want are available in an out-of-the-box model within a few months. Which model should you select?
Keep it simple. Go with the hyperscaler that has already been vetted and approved by your CISO and security team. You really can’t go wrong with any of the large multimodal models developed or backed by Nasuni’s cloud partners. As I’m drafting this story, Google’s Gemini ranks at the top for the first time, but that may very well change by the time you read these words. Amazon-backed Anthropic’s Claude and Microsoft 365 Copilot are just as brilliant. In fact, if you’re already an Office 365 shop, the choice may be fairly simple.
Yes, the costs are real, and the capabilities are expanding, so you will have to track both the developments in the field and the actual impact on your end users and organization to ensure you are backing the right tool and extracting enough value. This isn’t easy work. Tracking this space is confusing, even overwhelming. Unless you’re using AI on a regular basis you don’t see how fast it’s moving. But I’d caution against waiting any longer. You are not going to get a sense of what your people can do with these tools, and how your organization as a whole can benefit, until you officially adopt them as an enterprise. The longer you prevaricate the longer it will take to develop the muscle memory and understanding that these new breeds of tools require.
If you are a Nasuni customer, contact your account manager to discuss setting up an AI strategy session to see how we can help.