AI’s Growing Pains: Enterprise Lessons from Two Years of ChatGPT
Nasuni’s Jim Liddle reflects on two years of ChatGPT and what he predicts for the future of artificial intelligence.
October 29, 2024 | Jim Liddle
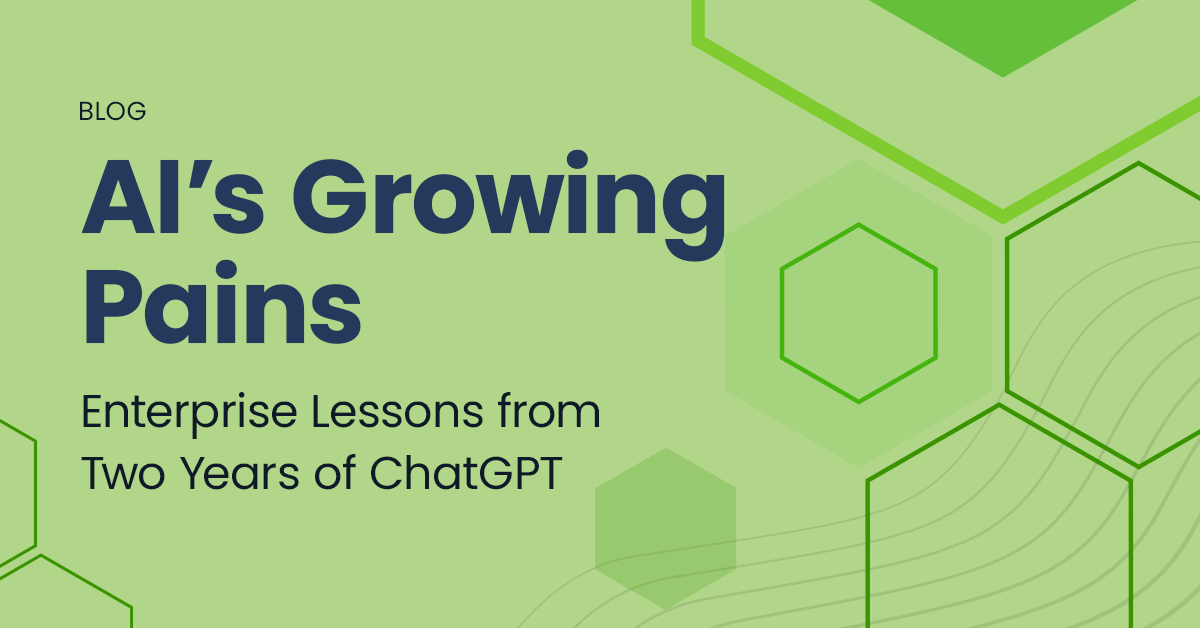
From its debut as a novel chatbot to its current status as a catalyst for innovation, ChatGPT has reshaped our expectations and sparked the creation and rapid evolution of many other capable artificial intelligence tools with applications ranging from profoundly impactful to entertaining.
GenAI has altered my working life by making me more productive and effective in my day-to-day job, yet marketing-induced confusion is rampant, too. Before ChatGPT was introduced in November 2022, AI was largely seen as a specialized tool, accessible primarily to tech giants and researchers. ChatGPT changed this overnight, instantly democratizing access to advanced language models and sparking widespread interest in AI capabilities. As we approach the second anniversary of ChatGPT’s public release, however, we find ourselves in an interesting phase, particularly in regard to enterprise adoption.
Once you filter out the hype and focus on the actual business impact, it’s clear that widespread enterprise adoption is still in its early stages. Based on the conversations I’ve had with enterprise leaders across our customer base, the current landscape of enterprise AI adoption is defined by 4 trends.
1. Cautious Exploration
Many enterprises are investigating potential use-cases and running pilot programs rather than fully integrating artificial intelligence into their core operations. This is wise for several reasons, but I wonder if the solutions providers themselves are partially to blame for the slow pace of adoption. Never mind those mind-numbing AI commercials. The tech giants and leading AI providers are releasing so much, so quickly, that it’s very difficult for anyone outside their walls to keep pace.
I’ve sat through meetings with enterprise IT leaders as AI solution providers pitch their tools and it’s a waterfall of technical information and terminology. Most IT leaders don’t want to hear about foundational models and fine-tuning. They want enterprise-ready tools that will solve their problems or drive efficiencies.
Instead of experimenting with the latest and greatest tools, enterprises are often drawn to solutions such as AI-enhanced chatbots. This is where AI starts for most enterprises – with familiar, easy-to-understand technology and a clear value proposition.
2. Identifying Use-Cases
We’re seeing pockets of adoption in areas such as customer service chatbots, code assistants, content generation, and data analysis, but full-scale deployment across business processes is not yet the norm. This is understandable. The whole ecosystem is moving so fast. We’re in a hype-cycle-type phase, and it is unclear whether we are advancing on to the next stages, or if the madness will continue for a while.
I suspect the emerging use-cases will be of a different sort than the intelligent chatbots — those that provide a much more enterprise-level function. If you deploy an effective intelligent chatbot that taps into your knowledge base through RAG, then you will reduce by a small percentage the number of customer services representatives required. On the other hand, the code assistants, content generation, and data analysis tools augment the capabilities of your employees. I’m certainly faster as a programmer because I no longer have to waste my time writing basic, boilerplate code.
This is the model I suspect will become more prevalent across the enterprise: Humans at the center, massively AI-augmented across different tasks. This is already happening in a subtle, below-the-radar manner, but I suspect that eventually it will become the enterprise-approved norm.
We will also start to see task-oriented AI agents that autonomously focus on a very narrow task but do it well and at scale. These may end up being so discrete and packaged in a way that we don’t even associate them with artificial intelligence.
3. Challenges to Adoption
Part of the challenge for the enterprise is that it takes a while for security teams to vet new tools and systems. Often, an organization’s internal datasets are in nowhere near any state to be exposed to AI. They need to be consolidated, curated, and secured.
Enterprises face additional hurdles such as data privacy concerns, integration with existing systems, and the need for AI-specific expertise. A tremendous amount of work here remains to be done.
4. AI Strategy Formulation
Right now, I would say that AI deployment across the enterprise is very mixed. Some companies and individuals within enterprises are moving at the speed of light. They’ve found a way to incorporate general or industry-specific artificial intelligence solutions into their organizational workflows and have either proven the impact or laid out a very clear plan to achieve measurable results. These organizations are the exception. Generally, prioritization of security and privacy makes it difficult to roll out advanced new technologies at a rapid pace.
As this space evolves, I’d suggest formulating and honing your AI strategy, both as an organization and as an individual. At the enterprise level, you could simply view AI as a way toimprove sales or cut costs, but that will limit the potential impact. Instead, think of how you can become an expert today so that you can use AI to be more productive and become more efficient at what you do. Immerse yourself in these technologies so that you and your organization have the skillsets and familiarity to capitalize when these tools really do catch fire from an enterprise readiness standpoint.
Exactly when that happens depends on how the tech giants and AI startups shape and steer these solutions in the next two years. I will address that subject in my next post. In the meantime, start experimenting and exploring if you haven’t already. These tools are truly getting better every week.