Can AI Be Leveraged In A More Sustainable Way?
Nasuni Chief Evangelist Russ Kennedy addresses how AI services can be used in a more environmentally friendly manner.
December 17, 2024 | Russ Kennedy
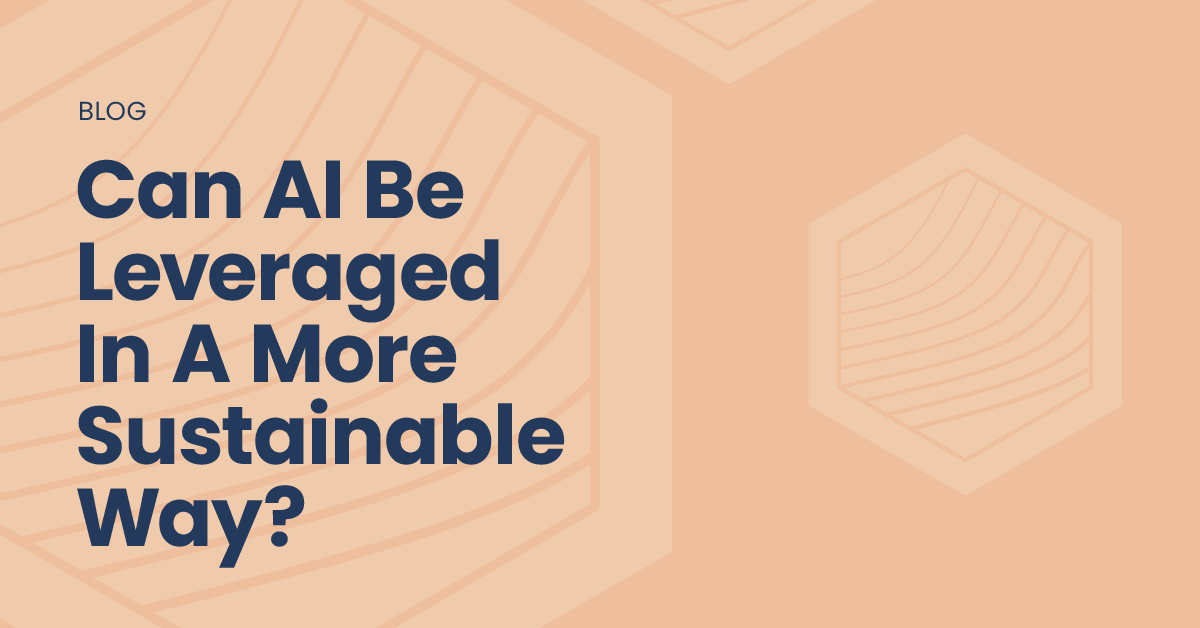
The evidence for AI’s energy appetite is mounting quickly. Advanced AI models like ChatGPT, Gemini and Claude depend on the compute capacity of vast data centers, and the graphics processing units (GPUs) required to run these models demand anywhere from four times to 15 times more than the traditional CPUs that do most of the computational work inside. According to the International Energy Agency (IEA), a Google search that makes full use of AI services, including an LLM-powered summary of scanned pages, consumes 10 times as much energy as a traditional query.
Training the foundational models behind the latest AI business tools is becoming increasingly energy-intensive, expanding by four to five times per year. It’s no surprise, then, that the IEA estimates the electricity consumption of global data centers could double by 2026.
We can’t continue on this path—especially not on a planet suffering from intense heat, droughts and extreme weather events due to anthropogenic climate change.
The hyperscalers have set ambitious goals to reduce their reliance on traditional fuel-powered electricity and fresh water used to cool data centers. But these sustainability efforts have veered off track due in part to the increased focus on energy-intensive AI and the rapid expansion of data centers to meet demand for cloud services. Amazon reports that they have met their energy-neutral impact goals, but Google and Microsoft both admitted to a spike in emissions in their latest sustainability reports.
As technologists and users, we have to do better, and I believe we will, but we need to realign our priorities and strategies. Here are five possible ways to do that.
1. Deploy advanced AI tactically.
If an inch of snow falls on my front steps in the winter, I grab a shovel, not a snowblower. Similarly, we don’t need to deploy fantastically advanced AI engines for every task. The Gemini-powered summaries that appear at the top of some Google searches are a good example of selective application.
If I search for something simple and specific, then some old-fashioned links to pages turn up. But if I broaden my query, an AI summary generated from a list of relevant pages appears at the top. Even here, however, the use of an energy-intensive large language model is questionable. I wonder whether this level of intelligence is necessary, given how effectively standard search has functioned for years.
2. Develop smaller models.
Typically, I write and revise my columns in Microsoft Word. This program has certainly advanced over the years, but its computational demands have grown as well. The first version of MS Word, released for Windows back in 1990, required 640K of RAM. Today, PC manufacturers suggest having more than 4GB of RAM available. Yes, the modern version is better, but is it 6,400 times better?
We can’t let enterprise AI models follow the same path. The next generation of tools should not only be applied tactfully but designed with energy efficiency in mind. Context window size and performance benchmarks should not be the only measures of progress. We need smaller, more efficient language models that are sized appropriately for specific tasks and use only the compute needed to generate impactful results.
3. Advance sustainable computing.
This should come as no surprise, but AI itself can help rebalance this energy deficit if put to good use. Researchers and companies could use AI services to reduce losses as electricity is distributed throughout the power grid, uncover new and more sustainable materials for use in data centers or develop more energy-efficient chips.
Google claims that one of its new chips is 67% more energy efficient than previous models and that they have identified changes in their processes that can reduce the energy required to train a model by 100 times. This is the kind of work we need to continue.
4. Explore novel renewables.
As the industry’s energy demands grow, we may need to look beyond wind and solar to meet our expected demand with alternative sources of green power, including geothermal and nuclear. The latter is a controversial technology for good reason, but the industry has also been designing plants the same way for many decades. Small modular reactors may be a potential solution, along with novel approaches like the Bill-Gates-funded startup TerraPower, which aims to have a smaller, safer nuclear plant operational in Wyoming by 2030.
5. Sharpen regulations and oversight.
Given the challenges the world faces around climate change, a technology that demands so much energy and water should be regulated. We should not leave it up to companies to decide how much electricity and freshwater they consume—not when these are both limited resources. There must be reasonable boundaries and controls that balance the energy needs of hyperscalers with those of the surrounding communities and countries that host their data centers.
The fear of AI services typically centers around superintelligent machines developing their own goals. While the timeline associated with this potential threat is uncertain, the risk of AI’s thirst for electricity is imminent, and we can’t bury our heads in the proverbial sand. We can’t simply marvel in wonder at the capabilities of these models without judging them by their impact on the grid. AI’s electricity problem is happening now, and as enterprise leaders, we need to address it now before it gets worse.